
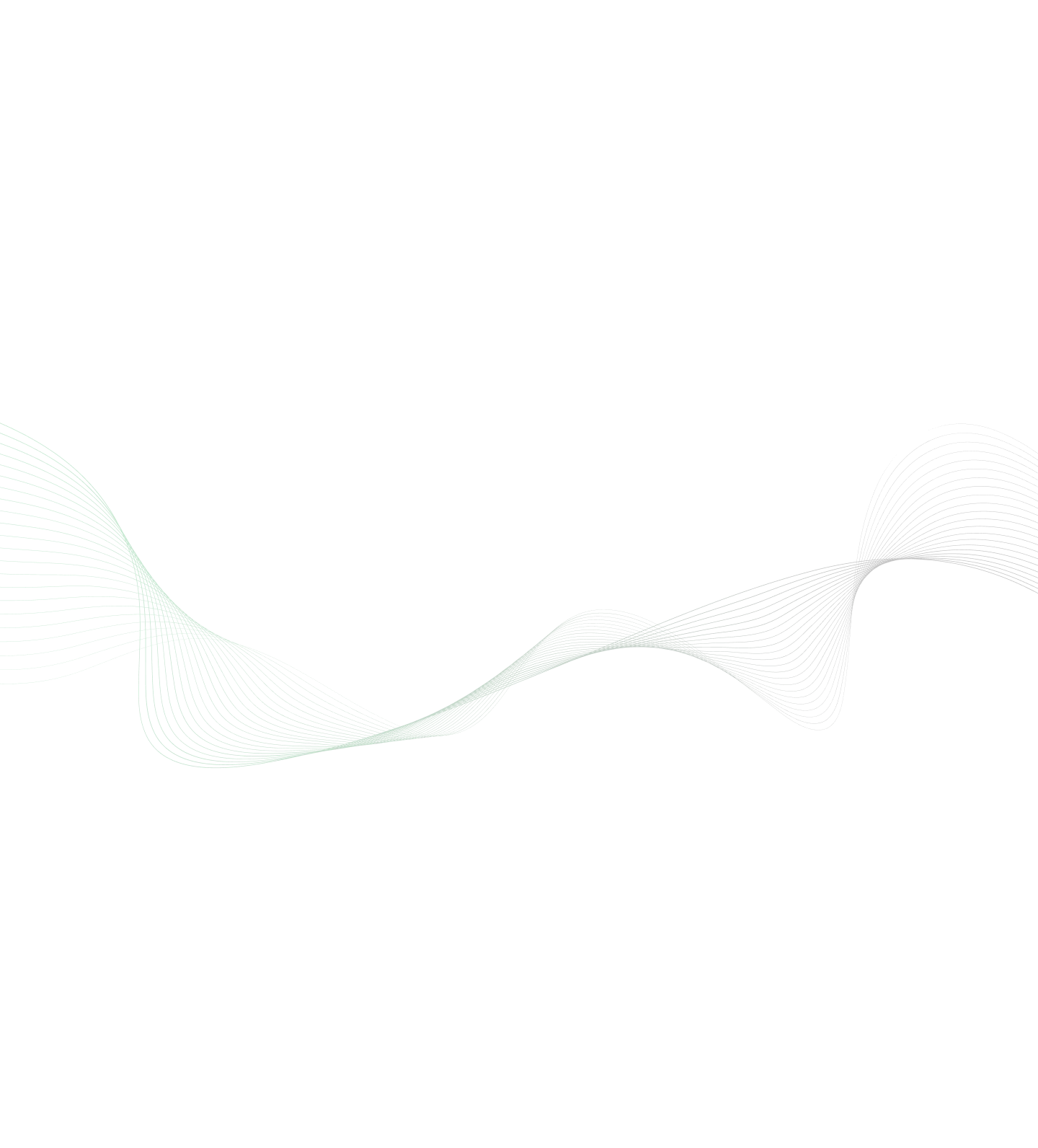
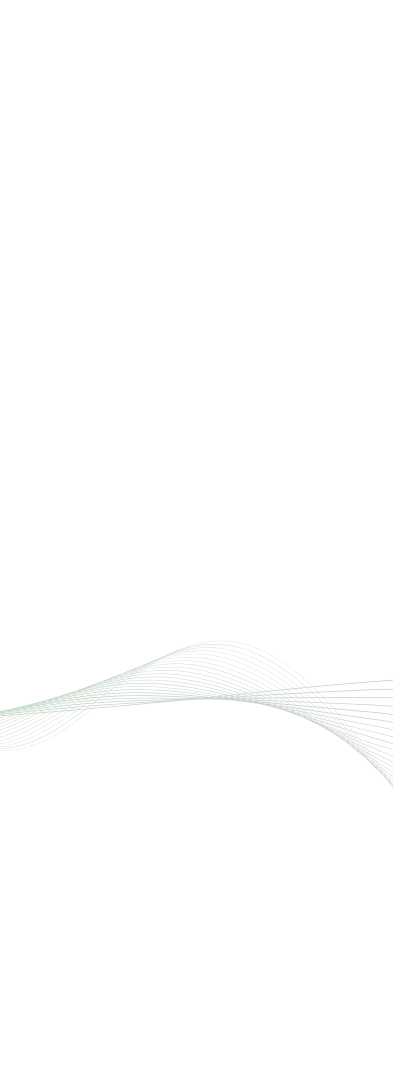
In recent years, an ever-growing number of organizations have been relying on data analytics to drive their decision-making processes, and understandably so. With a robust analytics framework in place, they can leverage their available data and make informed decisions.
However, as it stands, these organizations face multiple challenges in their path toward practicing "mature" analytics. Over 60% of companies cannot leverage their enterprise data for their business decisions. According to the MicroStrategy Global Analytics study, employees waste over 60% of their working hours trying to gain access to relevant data.
So, despite the growing value of analytics, insight-based companies are still fewer in number than those that have not adopted an analytical approach.
In summary, the need for analytics maturity arises from the growing recognition of data analytics as a strategic asset for businesses. By addressing the challenges associated with data utilization and adopting a mature analytics approach, organizations can unlock the full potential of their data, make informed decisions, and gain a competitive advantage in today's data-driven world.
In essence, analytics maturity is the level of progress that any company has realized in its journey toward data analytics. Here is everything you need to know about analytics maturity and its business benefits.
What is Analytics Maturity?
The analytics maturity model is a series of stages that represent how organizations evolve in their ability to leverage data for analytical purposes. This model determines the stage at each company should use its resources to extract value from its data.
As the term "maturity" suggests, the analytics maturity model indicates the organization's journey toward data evolution. Traditional analytics were based on qualitative or quantitative reports. This is insufficient to understand the data analytics capabilities of any organization.
Of course, for any data-driven organization, data analytics is now an essential capability. According to Boston Consulting Group research, the growth rate of data maturity has almost doubled from 8% to 15% since 2018.
Despite that, companies often face challenges in strategizing, implementing, and evaluating their data analytics projects. Among the major challenges, it's difficult to determine if companies are investing properly in data analytics and whether these investments are giving optimum returns.
The analytics maturity model is designed to solve these challenges. But the question is: how do organizations use the analytics maturity model? To answer that, let's understand the five stages of the analytics maturity model.
Five Stages of Analytics Maturity
To achieve analytics maturity, organizations progress through five stages, as described below:
No Analytics
This is the initial stage where the company does not possess any analytical capabilities. At this stage, the company collects data for effective reporting but does not perform any data analysis using technology tools. In this stage, business decision-making is based on individual intuition, industry experience, or a comprehension of the prevailing market trend.
An example of a company without analytics is a start-up business or a newly established business enterprise.
Descriptive Analytics
This is the second stage of the analytics maturity model. With this capability, organizations can understand their past and current developments with answers to questions like "What happened?" or "How many?"
At this stage, organizations can retrieve data from multiple sources, including case studies, customer surveys, and observational data. Most of this data is siloed and, thus, not easily accessible to business users. Hence, data does not drive most business decisions.
For instance, data management at this stage is based on manual spreadsheets, low-level ERP systems, or CRM systems. A successful case study is that of Portland State University, which used descriptive analytics to optimize its campus management and generate multiple reports.
Diagnostic Analytics
Also known as traditional analytics, diagnostic analytics is the third stage in the analytics maturity model. With this analytical capability, organizations can analyze and interpret historical data by detecting the relationship between different variables. It effectively answers the question, "Why did it happen?"
At this stage, data mining is deployed to discover data patterns and extract information from massive data volumes. Organizations also create and track multiple KPIs to improve their decision-making capabilities. In certain instances, organizations develop and add data lakes to their data pipeline and business intelligence framework.
Among the real-life case studies, Orby TV leverages BI and data analytics to manage their data and support data-driven business decisions.
Predictive Analytics
At this stage, organizations have the capability to generate accurate forecasts and predictions based on the outcome of their actions, events, and market trends. With predictive analytics, organizations can finally answer the question, "What will happen in the future?"
More profoundly, at this stage of analytics maturity, organizations can process and analyze both current and historical data to create data models and simulations. Employees also have real-time access to high-quality data to build customized reports based on their requirements. Organizations usually hire a team of data scientists to build predictive data models.
Among real-life examples, a sports retail company was able to predict market demand based on weather data and optimize operations. Likewise, platforms like PayPal can predict customers' buying intentions based on their feedback.
Prescriptive Analytics
This represents the final stage of the analytics maturity model. At this stage, organizations can deploy machine learning algorithms to automatically prescribe the next course of action. With prescriptive analytics, organizations can find answers to questions like "How can we address this problem automatically?"
Prescriptive analytics is the stage of automated analytics that supports decision-making by providing the best recommendations on the next course of action. Some successful examples of prescriptive analytics are companies like Amazon and Netflix, with their advanced customer analytics and recommendation engines.
How to Improve Analytics Maturity
Improving analytics maturity benefits organizations by enabling data-driven decision-making, identifying trends and customer behaviors, optimizing operations, and fostering a culture of innovation. It provides a competitive advantage in the data-driven business landscape. Here are some actionable steps organizations can take:
- Determine Your Current Maturity Level
The first step is to determine your current level of analytics maturity based on how your organization uses its data. Also, determine your data sources, technical tools for data analysis, and who has access to your data.
- Locate Other Available Data Sources
Next, you must research which other data sources are available both internally and externally. For example, create KPIs and metrics to collect customer feedback and monitor app performance.
- Focus on Metrics That Provide Actionable Insights
Focus on metrics that provide actionable insights by answering questions like "why" and "how" instead of simply answering "how many." This enables you to understand your business processes and customer behavior.
- Invest in the Right Data Analytics Technology
Finally, invest in technology tools that enable you to extract valuable insights from data. For instance, data visualization tools are the right investment for organizations with many non-technical or business users.
Conclusion
In today's data-driven environment, data is the lifeblood of every successful organization. To improve their data analytics capabilities, organizations must first understand the analytics maturity model and their current level of data maturity.
At Xoriant, we understand the business value of effective data management for our customers. We offer a host of services in data management, consulting, and solution development.
Check out our related PDF: XSVF
Want to improve your organization's analytics maturity level?