
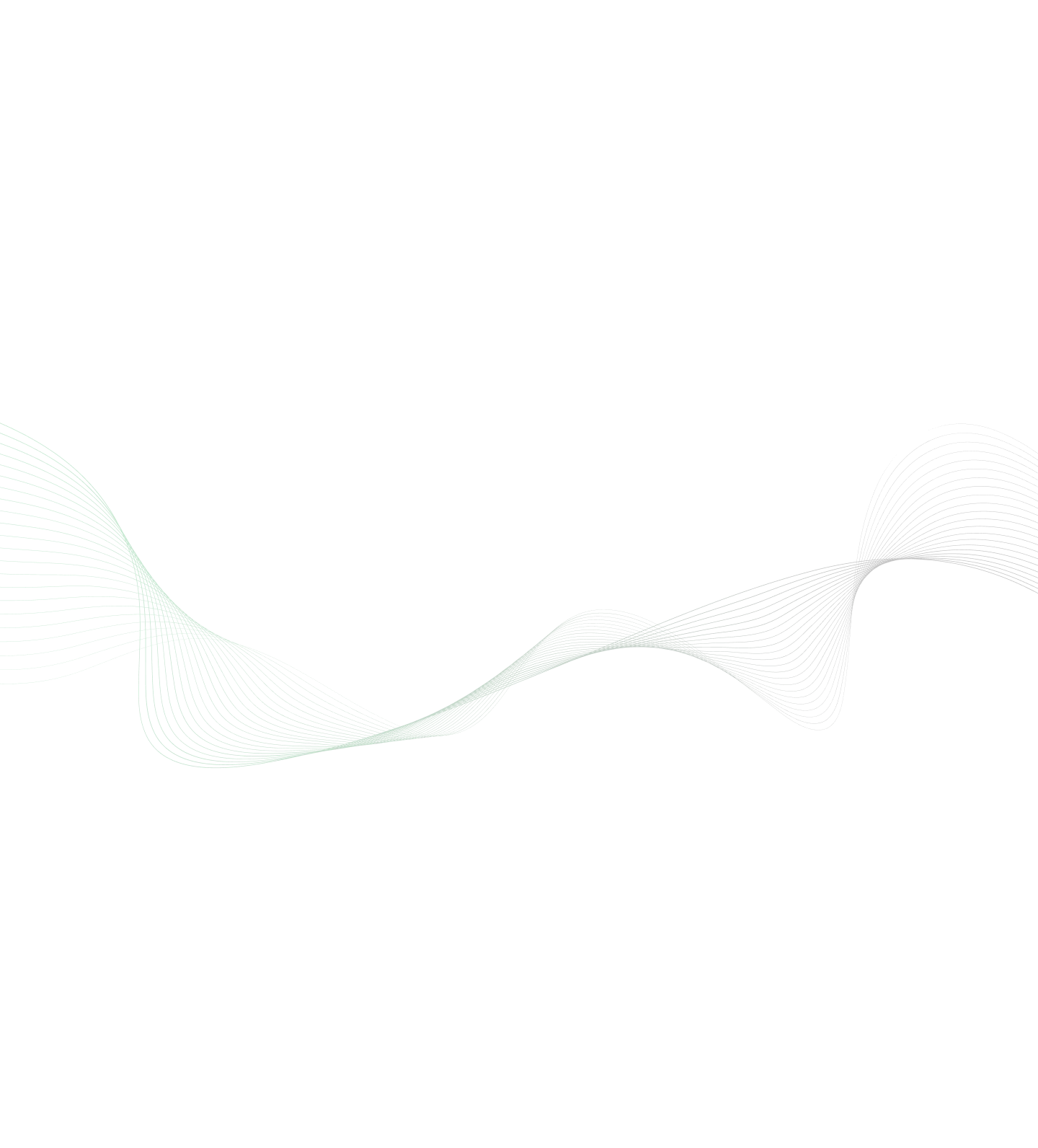
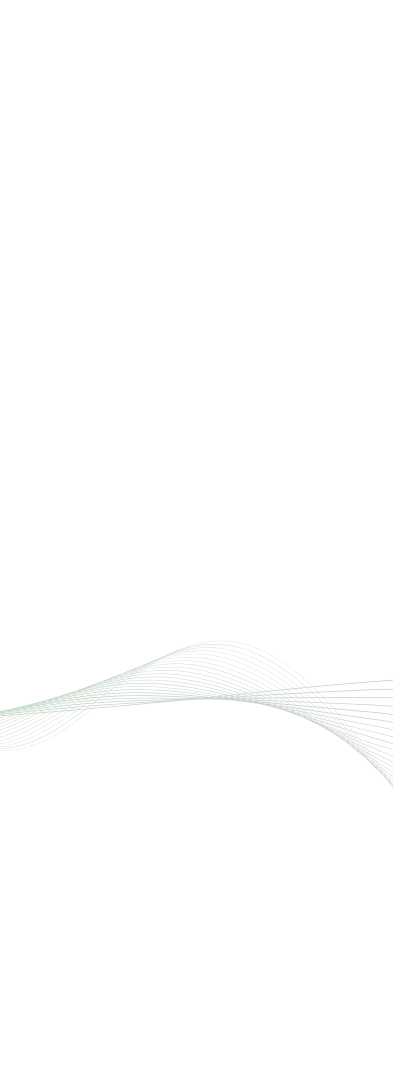
As enterprises shift their workload to the cloud environment and use more data for their businesses, the terms Extract, Transform, Load (ETL), and Data pipeline are sometimes used interchangeably to imply data movement.
But let’s look at what these terms really mean and how enterprises can leverage them to improve their business.
Data Pipeline
Data pipeline implies that the stored data is moved from one system to another through a set of processes such as data migration, duplication, and filtering. A data pipeline is an umbrella term used for moving data.
ETL
ETL is a type of data pipeline. It is more specific as it involves a set of processes to extract the data from a source such as a database, transform it into an intelligible format, and load it into another location such as the cloud.
In terms of similarities, data pipeline and ETL essentially do the same thing. They move data from one source to another to facilitate quick decision-making. It matters a lot in fast-moving industries like banking, as they have to process millions of transactions in minutes.
However, there are a few differences that enterprises must know.
What’s Different Between ETL and Data Pipeline?
Terminology
As the terminology implies, ETL involves the transformation of data. It transforms raw data that’s extracted from the source into a structured format before loading it. It provides the user with usable data that can be easily interpreted and used for data-driven decision-making. A data pipeline is a broad term that does not necessarily involve data transformation. Sometimes the data pipeline process doesn’t end with loading the data. It could also initiate new workflows and processes.
Objective
The primary purpose of the data pipeline is to store and move high volumes of data in real-time to help enterprises perform predictive analysis and make more accurate decisions. ETL has a more nuanced benefit because of its ability to transform raw data into a structured format. It helps the enterprises make quick and better data-driven decisions.
Data Movement
Data pipelines move the data in real-time with streaming computation, i.e., it is computed and moved as soon as data is generated at the source. It also runs in batches, i.e., it moves a high volume of data at regular intervals. ETL moves data in batches during a fixed schedule.
How can Enterprises Leverage ETL and Data Pipeline to Improve Business?
Good quality data is essential to running a business successfully. ETL and Data pipelines help enterprises move data from one source to another and ensure that the data analysts receive good quality data for analysis. It helps streamline the operations and improve efficiency. This is particularly important in banking, where users deal with highly sensitive data every minute. Any error, lapse, or delay in processing data could lead to heavy losses and a bad customer experience. Let’s look at how ETL and Data pipeline can benefit enterprises, especially the banks.
Real-Time Analysis
Most enterprises use legacy tools and processes to process data. Since most tools do not have the capabilities of real-time processing data, enterprises had to rely on historical data to predict trends and make decisions. In a fast-moving world where trends change quickly, enterprises cannot take that risk. They need access to real-time data to make accurate decisions. That’s where data pipeline and ETL can play a role. As the data is processed at the source and moved in real-time, enterprises don’t have to rely on old data to make decisions. They can use the latest updated data for analysis.
Intelligent Decision-Making
Normally, data is available in a raw and unstructured format. It is almost impossible for data analysts to make sense of it and gain any actionable insight from it. As ETL helps enterprises transform data into a structured format, analysts don’t have to spend too much time understanding it. They can make intelligent decisions quickly. Banks normally use data pipelines and ETL to detect frauds and take timely measures to avert risks. ETL also checks the credibility of data to ensure that there are no duplicate or false data. They reject such data, so only true data is sent for analysis.
Facilitates High-Frequency Data and Event Streaming
Several fintech companies, such as Revolut and Monzo, use ETL and data pipelines for high-frequency data and event streaming. It is also used in retail banks to update account balances in real-time, and process payments. It eliminates the need for lengthy procedures such as doing accounting based on the double-entry system.
What’s Next for ETL And Data Pipeline?
Banks and other enterprises have replaced legacy tools and processes with ETL and data pipelines to process data faster and make better decisions. While ETL helps banks and enterprises to stay aligned, they need to evolve further to meet the increasing demands. For example, it takes approximately 15 minutes to extract, transform, and load the batch of millions of transactions. As the volume of transactions increases, banks will have a tough time scheduling and transforming the batch. Hence, banks and enterprises could consider using automated ETL solutions to efficiently manage the large data volume in real-time and align the business with the changing customer demands.
ETL Framework for Regulatory Data Processing
Xoriant BFSI team collaborated with a global diversified financial service holding company to set up ETL for data processing which was used for regulatory reporting and analysis. We combined engineering rigor with next-generation technology expertise to build them a reusable, configurable and scalable framework. The data transformation service framework developed helped the client reduce 75% storage costs and delivered 70% faster data ingestion cycle than ETL.
Looking to save on data costs?