
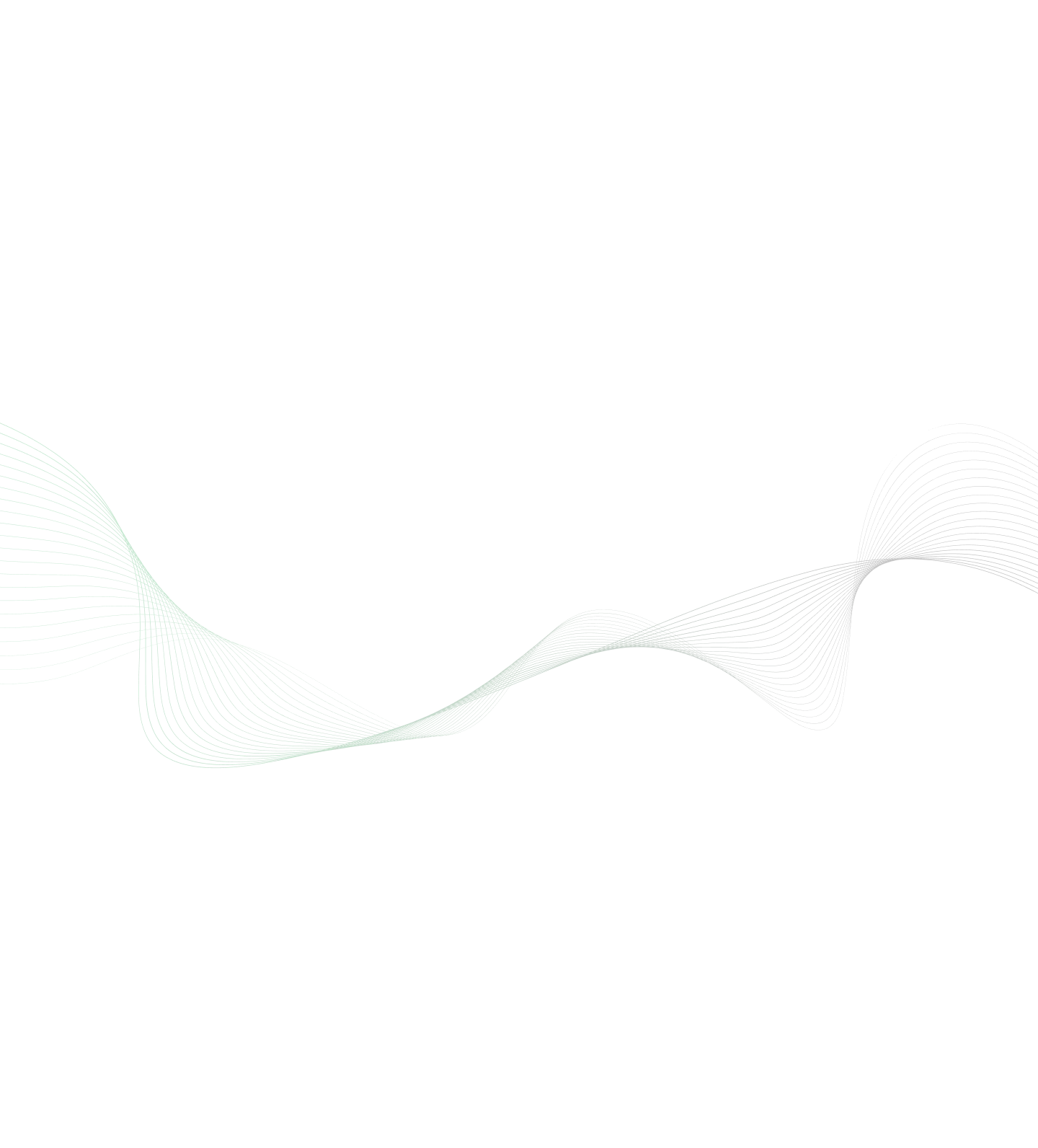
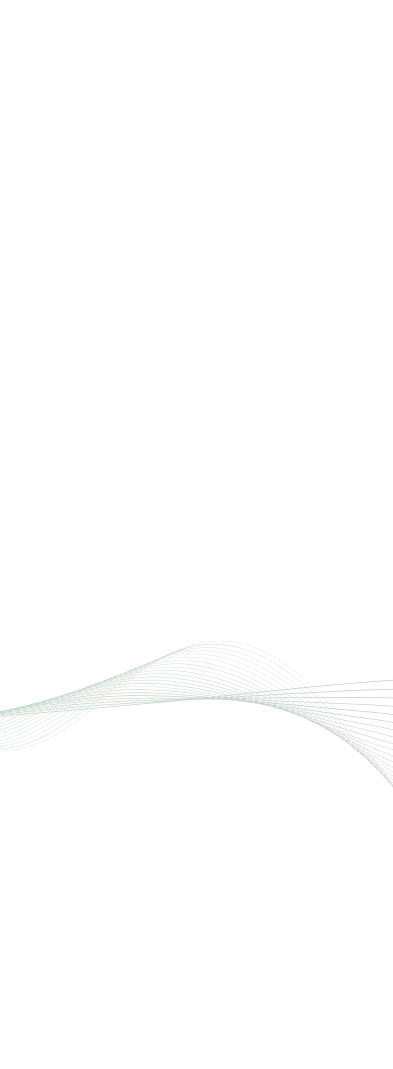
Introduction
Every organization today relies on data for decision-making and operations. In a dynamic and constantly evolving business landscape, data is more than just a collection of facts. It is a treasure trove of information and the lifeblood of modern businesses that allows them to propel into the future and excel. Informed decision-making through the utilization of data-driven strategies lies at the crux of successful enterprises.
However, a streamlined approach to data management is required to collect, store, clean, process, and use the massive volumes of data generated every single minute. Organizations can no longer take ad-hoc approaches to data management or have individual data architects and engineers work on data sets for departmental needs. To ensure effective data management and use, they require a comprehensive strategy with broad involvement and support from C-suite.
A data strategy aids in outlining the long-term objectives of data usage within the enterprise, coupled with policies and procedures that support those objectives. Prioritizing data strategy helps organizations distill intricate datasets into actionable insights and align data and business goals. Enhanced data proficiency accelerates the journey from data to decisions, setting organizations apart from the competition.
Comprising five critical components, such as data, tools, analytics techniques, collaboration, and documentation, a data strategy ensures the proper collection of data from various sources. Using the right tools and analytical techniques, a data strategy aids in the accurate processing of large data sets and generates critical insights that help decision-makers make the right decisions. The right data strategy KPIs also encourage frequent collaboration between various stakeholders as well as the documentation of policies, roadblocks, opportunities, and more.
Organizations that drive efforts toward prioritizing data strategy can ensure efficient data collection, integration, and storage. They can improve the quality of their data sets and make high-quality business decisions with proper data cleaning and preparation. A strong data strategy also helps in optimizing data pipelines for handling large volumes of data, while streamlining processing and enabling faster analytics and insights.
Understanding Organizational Objectives
Organizations are increasingly relying on data to base their decisions. But most of these decisions are taken in silos, which impacts consistency and performance. Without an overarching data strategy in place, it becomes difficult for organizations to unlock the true potential of data. When organizations have problems aligning data and business goals, it can lead to several issues.
- Lack of alignment can impact the relevance of decision-making, as teams cannot accurately prioritize data-related tasks that have the most significant impact on business performance.
- Because there is no clarity or agreement on common objectives, there is a higher risk of investing in projects that do not contribute to the collective achievement of long-term goals.
- Poor alignment leads to wasted time, energy, and resources on activities that do not deliver tangible benefits.
- Investing in projects that fail to address business needs restricts organizations from demonstrating the true value of their data initiatives.
Prioritizing data strategy is a sure-fire way to maximize ROI by aligning data and business goals. It enables organizations to be extremely agile and adaptive, adapt quickly to shifting priorities, and respond quickly and effectively to market changes. When organizational goals underpin the data organization, it also leads to better cross-functional collaboration across various departments and functions within the enterprise.
To curate a data strategy that aligns closely with business goals, organizations must take several crucial steps. They must assess their current state of data maturity, get a broader understanding of the capabilities they need to acquire, and evaluate their existing tools, processes, and skill sets. They must plot out an implementation roadmap and develop an internal communication plan to ensure stakeholder agreement across the entire journey.
A data strategy that addresses organizational goals also requires deep involvement from various stakeholders. Building a team of people who set data policies, build data architectures, or simply use data for their day-to-day tasks—from data users to data analysts, business leaders to data engineers, and more—requires consultation while prioritizing data strategy. Their involvement plays a huge role in crafting a robust strategy, monitoring and measuring progress, and cultivating data culture and literacy.
Assessing the Current Data Landscape
Data has become the new fuel, powering the organizations of tomorrow and enabling them to meet the evolving needs of customers. But today, data is flowing through the business world like never before, with every device and process generating humongous amounts of data—from smartphones to wearables, IoT devices to IT systems, customer interactions to employee databases, and more.
In today’s data-obsessed era, the variety, volume, and velocity of data are constantly increasing. With new devices, new processes, and new systems surging, the data landscape has become extremely complex. Hundreds of different data sources now exist across modern enterprises, generating various formats of data at lightning speed.
Decision-making requires a quick and efficient analysis of this data. However, several challenges exist in ensuring the quality and accuracy of the data. The data that is currently available to organizations isn’t always clean. Simply feeding this raw data into analytics or machine learning algorithms can lead to several damaging consequences. Processing incorrect, biased, or incomplete data can result in inaccurate predictions, which can impact overall decision-making.
For instance, a wearable health device might generate thousands of terabytes of data each day, such as heart rate, steps taken, calories burned, sleep patterns, and more. However, a dashboard does not need to process or share all of this data with the user. Only clean, relevant, and updated information must feed into the algorithm for effective processing of health data. Not only does this ensure accurate processing of data, but it also speeds up decision-making.
Preparing and cleansing raw data and making it suitable for further processing and analysis is a key element of any data strategy. When done right, data preparation:
- Ensures that the data used for analytics produces reliable results.
- Helps identify and fix data issues before it is processed, thus minimizing the far-reaching consequences of wrong decisions.
- Avoids duplication of data as well as effort in preparing data for use in multiple applications.
- Helps consolidate similar data from different sources while removing data that isn’t needed and enriching correct data.
Organizations must adhere to specific policies and procedures to guarantee the use of only clean and accurate data for decision-making. They must collect, cleanse, and label raw data into a format that is easily understandable and analyzable by data analytics or machine learning algorithms. To form highly effective data sets, they must also annotate the data and ensure label quality, which, in turn, boosts the efficacy of data analytics models and frameworks.
However, most organizations have a very complex and cumbersome data landscape, with data trapped in a maze of disconnected systems. In a world that demands precision, these siloed systems and approaches to data management are akin to a treasure map without a compass. Overwhelmed by data silos, most organizations struggle with data quality, which not only impacts business results but also results in several missed opportunities.
Data silos also cause organizations to battle with real-time integration challenges, governance issues, and an inability to manage surging data sets. Manual management of growing sets of data hurts productivity and workforce performance. Inefficient resource utilization further adds to operational costs while also having adverse effects on customer experiences.
Leveraging Data for Competitive Edge
Organizations have long relied on data across various aspects of their business. From customer purchasing behavior to equipment performance, market trends to economic conditions, and more, while all of this data has enabled decision-makers to base decisions on historical evidence, gathering this information and using it to inform decision-making has traditionally been a slow process, restricted in scope, and tough to scale up.
With advancements in cloud and analytics technologies, organizations are in a far better position to use this data to enable a competitive edge. Modern analytics tools have the power to analyze massive volumes of data from multiple data sources simultaneously, thus accelerating the decision-making process. Using these tools, organizations can deep dive into various aspects of their operating environments and make decisions to outdo their competition:
- Consumer Insights: Customer data acts as a treasure trove of information for businesses. This data, when turned into insights, paves the way for greater customer satisfaction.
- For instance, an e-commerce user’s online behavior can be regularly tracked to analyze purchasing history, understand his/her current preferences, and deliver content or products/services with a higher degree of personalization. Such customization of offerings can allow businesses to better predict and meet customer needs, as well as enhance customer satisfaction and loyalty.
- Similarly, businesses can act on customer surveys, reviews, and feedback to identify areas for improvement and make essential tweaks to their products or services to enhance customer satisfaction levels.
- Market Intelligence: Enterprises that harness market data can achieve a greater edge over their competitors. Insights into market trends, opportunities, and threats can inform strategic decision-making for organizational growth and success, as well as enable data-driven competitive advantage.
- For example, a business looking to enter a new market can use competitor intelligence to get a clear idea of pricing and promotions, better plan its pitch to reach a wider audience, and stay a step ahead of the competition.
- Likewise, companies can use market intelligence insights to understand forecasts and predict future product trends. By foreseeing impending downturns or changes in customer demand, they can adjust their strategies ahead of time and gain a competitive advantage.
- Economic Trends: Economic trends such as inflation, growth, and geopolitical risks impact business performance in several profound ways. To make the right business decisions, organizations need to keep track of these trends and evaluate how they might impact demand and costs.
- For example, a company looking to launch a new product can quantify the influence of inflation on performance or profitability, be aware of the threats and weaknesses it might face, and capitalize on its strengths and opportunities.
- Similarly, companies can study the impact of economic fluctuations on labor market conditions and target job seekers with suitable skill sets. By identifying emerging labor market trends, they can better plan for recruiting, hiring, and retaining talent.
Implementing GenAI: Maximizing Data Strategy for AI Integration
Artificial Intelligence (AI) has redefined several aspects of business. By mirroring human actions, it has excelled in areas like speech and translation. However, the technology lacks creativity in writing, music, and art, which generative AI overcomes. From generating fresh ideas to optimizing algorithms, generative AI is revolutionizing the norm.
The growing buzz around Generative AI has propelled the excitement around data to grow tremendously. Leveraging large language models and advanced natural language processes, and deep learning capabilities, GenAI algorithms are revolutionizing how data is analyzed and decisions are made. By uncovering patterns and insights that are challenging for traditional analysis models, GenAI brings about a great level of automation and precision in daily tasks. Source: Xoriant
However, with Gen AI's rapid growth and rising customer and stakeholder interest, enterprises often find themselves struggling with many complexities and challenges. To unlock the full potential of GenAI and maximize the investments in such transformation initiatives, there is a pressing need to build the right AI integration strategies. Organizations must carefully evaluate and strategize for the successful adoption of Gen AI, whether it is for optimizing customer experiences, automating processes, or revolutionizing data analysis using advanced data analysis tools.
- Leveraging Advanced Data Analysis Tools with GenAI: Tweaking the existing data strategy to accommodate the capabilities of GenAI can help organizations harness the power of artificial intelligence for enhanced data analysis and decision-making. Using advanced data analysis tools with GenAI, decision-makers can analyze vast datasets and identify trends with greater accuracy. They can automate the data processing pipeline, streamline decision trees, and respond swiftly to changing market conditions. They can also receive recommendations based on a holistic analysis of historical data, current trends, and future predictions, enabling data-driven competitive advantage.
- Enhancing Predictive Capabilities: GenAI, when powered by quality data and successful data strategies, enables organizations to develop advanced predictive models. For instance, advanced data analysis tools underpinned by GenAI models and a strong data strategy can empower marketing teams to make superior demand forecasting or churn prediction decisions. Using demand sensing capabilities, these models can analyze accurate, relevant, and updated data across demographics, weather, price changes, and consumer sentiment, resulting in more accurate forecasting and proactive decision-making.
- Driving Innovation and Efficiency: Prioritizing data strategy also plays a huge role in enabling organizations to leverage GenAI for innovation and process optimization. Through the collection and analysis of good-quality data from multiple sources, GenAI can uncover market trends, customer preferences, and emerging patterns, providing valuable insights for innovation. By staying a step ahead of emerging trends and changes, organizations can innovate products, services, and business models, drive efficiency gains, foster continuous improvement initiatives, and stay ahead of the competition.
- Ensuring Ethical and Responsible AI Use: A well-defined data strategy that is fortified with robust governance policies also allows for the ethical and responsible use of AI. Such a strategy helps to mitigate the data privacy and security risks associated with generative AI, while also ensuring compliance with evolving regulatory requirements. The presence of quality data processes and standards ensures the accuracy, consistency, and reliability of decisions, as well as protection against unauthorized access, breaches, and misuse.
At the same time, to ensure successful GenAI implementation, organizations must rethink their data strategy and see how they can pave the way for seamless integration.
- Assessing use case suitability: The first step towards the successful adoption of GenAI is use case identification. This is critical to ensuring that identified use cases align with business goals and deliver tangible benefits. It also aids in optimizing resources and time, ensuring relevance, and maximizing business impact.
- Ensuring data readiness: Data lies at the foundation of GenAI training, the right data is critical to identifying patterns, making precise predictions, and generating valuable content. Once the use cases have been properly identified, organizations must then move on to identifying and preparing the data that will be fed into Generative AI models.
- Evaluating talent requirements: Successful GenAI adoption also necessitates the presence of a skilled workforce that can navigate its way around new trends and adapt the data strategy accordingly. While several existing skill sets will be relevant in building AI integration strategies, enterprises will also need to hire new skills or invest in reskilling/upskilling their existing talent to enable expertise in GenAI algorithms and models.
- Technology considerations: Integrating GenAI models into the existing data strategy requires organizations to have a comprehensive toolkit in place for model development, training, and deployment. From cutting-edge machine learning frameworks to cloud computing services, data processing tools, test environments, and more, underlying hardware that come together and enable AI models, organizations must make several critical choices to ensure their GenAI initiatives work in close association with their data strategy.
- Risk management: Effective GenAI adoption also requires organizations to address the many associated risks. These range from data security and privacy, explainability, ownership and responsibility, bias, and more. Proactively addressing these issues can enable businesses to fully leverage the benefits of Gen AI while mitigating the many potential risks that might emerge along the adoption journey.
Defining a Data Governance Framework
The success of modern businesses is often defined by how well they can collect, control, and monitor their data. Although reliance on data is constantly surging, understanding what data is and how best to use it to meet company objectives is still a hurdle for many businesses. Data governance best practices help outline the processes associated with managing the availability, consistency, security, integrity, and usability of data. A robust framework guarantees the reliability and effective use of data for business decision-making.
Data governance best practices play a huge role in managing an organization’s data assets. By delivering against prioritized business objectives, it helps unlock the value of data across the organization. However, any data governance program must be capable of solving business problems and delivering outcomes.
Defining a robust data governance framework requires organizations to identify business objectives, desired outcomes, key stakeholders, and the data needed to deliver these objectives. They must formulate several structures, rules, and processes to streamline potentially complex governance tasks and ensure the scalability, collaboration, and management of large data sets. They also ensure compliance with data-management standards and regulations, helping businesses have greater control over how data is administered and managed across the entire enterprise.
However, given that different organizations collect different types of data to run their operations, there are no one-size-fits-all data governance best practices. Such an approach neither fits the individual scope of different businesses nor does it have the agility to meet their unique needs.
If organizations want to respond to varying levels of uncertainty in today’s world, they need to take a modern and adaptive approach to data governance. They need to ensure accountability, trust, and transparency. A robust framework also helps in addressing evolving risks through frequent collaboration, education, and training. Such an approach enables the flexibility they need to apply different governance tactics to different business scenarios.
Here are the key steps involved in defining a successful data governance framework:
- Establish data governance policies: Formulating policies and guidelines to ensure effective management and utilization of data is the first step in defining a data governance framework. The organization uses these policies as a set of documented guidelines to govern, manage, protect, and use its data assets. The organization's data strategy should drive them, align them with business objectives, and regularly update them to keep up with evolving market trends and business needs.
- Define roles and responsibilities: When defining a data governance framework, it is critical to assign clear roles and responsibilities within the organization. Every level of the organization must identify key stakeholders, as any framework must facilitate collaboration. From data owners, who monitor and control data sets, to data stewards, who ensure data practices are in line with established policies and standards, organizations must also form a governance committee with members who assist in establishing, approving, and updating data standards and policies, as well as making key decisions when faced with data issues.
- Ensure compliance with regulations: Ensuring adherence to relevant data protection and privacy regulations is a key aspect of any data strategy. To do this, organizations must institute standards so that every data set is easily usable and accessible. Automating governance workflows and requests is a great way to ensure accuracy and reduce the burden on team members. Organizations must also continuously monitor the data organization to track metrics, identify outliers, work towards data quality improvement and use, and make changes to the governance framework as regulations evolve.
Data Collection and Integration
In the modern business landscape, data collection happens on multiple levels. Businesses regularly collect data on customers, employees, sales, and other aspects of their operations. Companies also conduct surveys and track social media behavior to get feedback from customers. Large and complex datasets from many different and unconnected sources overwhelm most decision-makers, leading to countless hours spent preparing and tying data together to provide a complete picture of the business.
Organizations can obtain the necessary information to analyze business performance and forecast future trends, actions, and scenarios through effective data collection techniques. However, with hundreds of data sources available, gathering the right data from the right sources for use in business decision-making is a crucial aspect of any data strategy. Here are key aspects businesses should consider while crafting effective data collection techniques:
- Identifying relevant data sources: Identifying and accessing data sources that are pertinent to organizational objectives is extremely important. To do this, businesses must start by having a clear idea of their data objectives. Identifying the type of data required to address specific problems is an essential initial step. They must also assess the resources they have available, including time, budget, skillsets, and tools, and then narrow down the number and type of data sources they can target for data collection. It is also imperative to evaluate the quality of sources and choose those that are reliable and relevant. Once organizations identify data sources, they must integrate disparate data sources to obtain a complete, accurate, and up-to-date dataset for data analysis. This helps break down data silos and enables the formation of a reliable, single source of governed data that businesses can trust. By creating a unified and comprehensive dataset, organizations can eliminate the risks arising from duplication, error, and dissimilar data formats while enabling decision-makers to get a 360-degree view of data from across the business. Proper integration also helps to increase data accuracy, fuel trust, and increase efficiency, paving the way for collaborative decision-making and data-driven competitive advantage.
- Ensuring data consistency and accuracy: Another crucial element of effective data collection techniques is taking measures to maintain data integrity and accuracy throughout the integration process. Making sure the data utilized for decision-making is accurate, current, and error-free significantly contributes to achieving the desired results. Proper validation, cleansing, and profiling of data ensure decisions and strategies are based on a solid foundation, minimizing the risk of negative consequences resulting from bad data quality. Regularly checking data for errors, inconsistencies, and inaccuracies and correcting them can lead to accurate decisions and improved operational efficiency.
Data Analysis and Insights Generation
Most organizations today are embracing data-driven approaches to drive their business forward. But siloed and traditional approaches create several inefficiencies that might take months or years to resolve. According to McKinsey, by 2025, nearly all employees will naturally and regularly leverage data to support their work. As the dependence on data continues to increase, there is a pressing need to take data analysis and insight generation seriously. Here are some factors to keep in mind:
- Implement analytics tools and techniques: To enjoy a data-driven competitive advantage, organizations must embrace advanced data analysis tools, technologies, and methodologies to automate basic day-to-day activities and focus on innovation, collaboration, and communication. Advanced analytics and custom visualization techniques can help turn complex data into actionable, easy-to-understand insights. With real-time monitoring and trend analysis, they can optimize processes for efficient operations and help foster a culture of continuous improvement, resulting in truly differentiated customer and employee experiences.
- Extract actionable insights from data: Advanced data mining, OLAP, and analytics techniques allow organizations to transform raw data into actionable insights that drive informed decision-making. They can embrace intelligent algorithms to foresee trends, mitigate risks, uncover opportunities, and get a deeper understanding of their data landscape. Using regression analysis, they can also unearth precise insights and hidden patterns and relationships in data. Dynamic visual frameworks can further aid in facilitating instant querying and interaction, ensuring swift, data-driven decision-making.
- Enable data-driven decision-making: To enable data-driven decision-making, organizations must enable seamless access to critical insights. Modern data visualization techniques can empower stakeholders with critical insights. Via intuitive dashboards and reports, they can convert complex information into comprehensive visuals and more easily identify trends and patterns in data. The right visualizations can also bridge the gap between technical and non-technical experts, enabling real-time access to visual frameworks, streamlining data resolution, and paving the way for strategic decisions.
Data Security and Privacy Measures
Today, data is essential to every company, providing a wealth of new opportunities and empowering businesses to make informed decisions and achieve desired results. However, this increasing reliance on data also makes organizations vulnerable to a barrage of data security and data privacy risks. Aging code, scalability issues, and frequent downtime allow hackers to infiltrate corporate networks.
Organizations that want to protect data from evolving cyberattacks and vulnerabilities must invest in various measures to ensure the right levels of protection, encryption, and authorization.
- Implementing data security protocols: Data security protocols help safeguard sensitive business and customer information from unauthorized access, breaches, and misuse. Organizations can choose from a range of data security protocols and measures across firewalls, authentication and authorization tools, VPNs, and more. They must also invest in advanced data encryption methods, secure application development practices, and robust cloud app security solutions to safeguard the business against even the most sophisticated threats.
- Ensuring data privacy compliance: In 2023, violations of the General Data Protection Regulation (GDPR) cost companies over 2 billion euros, more than the combined costs of the three previous years. To avoid the negative consequences of noncompliance, organizations must ensure adherence to data privacy regulations and safeguard individual privacy rights. They must regularly revisit their encryption, access controls, and anti-leak measures, conduct frequent data security audits to pinpoint potential risks, and craft custom strategies to keep data safe.
- Mitigating risks of data breaches: Data breaches can happen anytime, anywhere, and impact almost any organization. While it is impossible to prevent breaches from happening altogether, organizations can take several steps to proactively identify and address potential vulnerabilities and minimize the impact of data breaches. From storing data securely to creating strong security policies, embracing data governance best practices to periodically monitoring data usage, training and educating employees on the latest threats to properly disposing of sensitive data – these steps can strengthen the data foundation and minimize the risk of attacks.
Continuous Monitoring and Improvement
Continuous monitoring and improvement are an integral aspect of a robust data management strategy. It plays a vital role in maintaining the integrity, accuracy, and security of data. In today's data-driven landscape, organizations must constantly update their approach to data management and ensure the effectiveness of their data strategies.
Such monitoring can help businesses evaluate progress toward goals and ensure they are aligning data and business goals. It can also help detect issues early, make informed decisions, and maintain a competitive edge.
- Establishing KPIs for measuring data strategy effectiveness: The first step to enabling continuous monitoring is defining key performance indicators (KPIs) to assess the effectiveness of the data strategy. Identifying data strategy KPIs and metrics that align with the organization’s data objectives can aid in tracking performance, detecting bottlenecks, and planning mitigation steps. Metrics across data quality improvement, data governance, adoption rates, etc. are a great way to measure data strategy effectiveness.
- Implementing regular audits and reviews: Conducting periodic evaluations and audits to monitor data strategy KPIS and performance is equally important. Such monthly or quarterly reviews can help in offering a clear picture of how the business is achieving its data goals. It can also help summarize key challenges, trends, and areas for improvement and gauge adherence to necessary policies.
- Iteratively improving data strategy based on insights and feedback: As an organization needs to grow and the variety of data increases, businesses must also continuously refine the data strategy. Based on insights gained from monitoring and feedback mechanisms, they must iteratively improve their data strategy KPIs and ensure it aligns with new market trends, business requirements, and user expectations.
Cultivating a Data Culture
Driving data-driven competitive advantage isn’t just about crafting a data strategy or using the right tools. There is also a pressing need for cultivating a data culture where everyone in the organization works collectively to improve decision-making. People who value, practice, and encourage the use of data ensure its integration into every operation and decision, enabling the organization to tackle even the most complex business challenges.
- Fostering a culture of data-driven decision-making: A culture of data-driven decision-making helps enterprises align data and business goals. Cultivating a work environment that values data and uses it to inform decision-making processes is crucial to foster such a culture. It is also important to provide the workforce with seamless access to data and analytics so they can uncover insights with minimal guidance. While this can help accelerate business decision-making, it also enables the workforce to understand the value of data and its role in completing tasks with accuracy and precision.
- Providing training and education on data literacy: Data can be complex and extremely cumbersome to work with. However, this does not imply that only skilled data engineers and scientists should use it. To enable everyone in the organization to work with data seamlessly, organizations must offer training programs to enhance data literacy skills among employees. Empowering the workforce to understand, explore, and communicate with data in a meaningful way can go a long way in building confidence, ensuring informed decision-making, and driving ROI.
- Encouraging collaboration across departments for data utilization: Organizational silos prevent the realization of the true value of data. Promoting cross-departmental collaboration can help team members share perspectives and insights, establish common goals, and understand the significance of each data point. Encouraging collaboration across departments for data utilization also helps foster an environment where collective intelligence thrives.
Case Studies: Examples of Successful Data Strategies
Organizations with successful data strategies enjoy several profound benefits in comparison to those that don’t. Having the right data foundation not only improves the quality and accuracy of decision-making, but also allows organizations to drive better growth and profitability, outdo the competition, and enhance the customer experience. Prioritizing data strategy also aids in enhancing operational efficiency, reducing costs, and improving the organization’s ability to respond to change.
Case Study 1
A leading car manufacturer was always looking to create new digital and connected experiences by enabling data-driven decisions. While the group has long been generating historical, real-time, and predictive insights from sensors in vehicles, operational systems, and data warehouses, they needed to bridge silos and accelerate the pace of innovation.
- Crafting and implementing successful data strategies allowed the manufacturer to better understand data and make accurate decisions.
- By re-architecting and moving the on-premises data lake to the cloud, the manufacturer could anonymize data from vehicle sensors and other sources across the enterprise.
- Having an environment that can scale to meet the needs of data engineers empowers the car manufacturer with the autonomy and agility required to continue to innovate.
- A modern portal enables analysts to identify trusted datasets using an advanced search algorithm and easily query data to produce new insights.
- Consumers can have real-time access to vehicle telemetry across speed, location, temperature, brake levels, engine status, and more.
Case Study 2
A professional sports league labor union was struggling with data management. While the league relied on a CRM system to store player data, contracts, marketing details, and financial data, it was facing challenges with data storage, performance, and efficient utilization of resources.
- The league could optimize the data for analytics and reporting by designing and developing a robust data strategy and efficient ETL pipelines.
- Semantic models for BI reports and dashboards enabled the creation of clear and concise data visualizations that encouraged data-driven decisions.
- By moving on-premises data to the cloud, the league experienced a notable reduction in CRM storage space cost.
Data Strategy Trends 2024
In the coming years, data and analytics will bring as many opportunities as challenges. To make the most of the opportunities while addressing challenges as they arise, enterprise leaders should drive efforts in prioritizing data strategy, measure and monitor data strategy KPIs, and embrace best practices for data and analytics to maximize the value of data.
Additionally, as generative AI continues to evolve, companies of all sizes will explore the numerous opportunities this technology presents. According to a Forrester study, 89% of AI decision-makers say their organizations are expanding, experimenting, or exploring the use of GenAI. But for generative AI to deliver its magic, data and analytics leaders must enable the people, processes, and platforms to set the stage for success.
Leaders must embrace data platforms and data quality improvement practices to support these evolving LLMs and upskill their workforce to deliver value with new AI technologies, as the outputs from AI technologies are only as good as the data they input. Keeping these in mind, here are some data strategy trends for 2024 to look for, as demonstrated by Forrester in its Predictions 2024 study:
- Vector database adoption will increase by 200% to support GenAI initiatives: As everyone looks to exploit GenAI advancements, the demand for vector databases is poised to grow. Vector databases store data in mathematical constructs with built-in search capabilities, in contrast to traditional databases that organize data into static tables, rows, and columns. These databases will deliver optimized and relevant results, especially with complex data sets such as images, audio, and video, enabling GenAI applications to run complex analyses quickly and efficiently.
- As generative AI becomes increasingly ingrained in organizational DNA, the upcoming year(s) will see an increasing number of employees receiving prompt engineering training. Such training will enable citizen employees to structure the data being fed into GenAI models while enabling them to communicate more effectively with these models. Organizations will also be seen investing in data/AI literacy programs to bridge the prompt engineering skills gap and support them in building AI integration strategies and leveraging generative AI consistently and safely.
- Data quality improvement will enhance AI/ML models’ accuracy by 20%: Organizations have always understood the significance of feeding good quality data to analytics algorithms. But in this day and age of AI, the importance of data quality has grown manifold. Since AI models are only as good as the data they feed on, organizations will be seen driving efforts toward data quality improvement, so their AI models can accurately identify underlying patterns, minimize the risk of biased or erroneous results, and boost prediction accuracy.
Conclusion
In today’s digital world, data has become a competitive differentiator. Companies that can use data to their advantage are the ones truly enjoying success. However, given the vast number of data sources and the numerous complications involved in data collection, storage, analysis, governance, and use, prioritizing data strategy is vital.
A data strategy helps establish long-term objectives for the use of data in any organization. From how it is collected, cleaned, and processed to how it is used, by whom, and for what purposes, every modern business today needs to track data strategy KPIs to ensure the data that they base their decisions on is accurate, well managed, and used effectively.
By cultivating a data culture and using the right tools and methodologies, organizations can unearth critical insights into growing volumes of data. With modern data visualization, strategic decision-makers can fully understand analysis outcomes and make accurate and consistent data-driven decisions. By embracing data governance best practices, they can enhance accountability and trust while mitigating risks as they emerge.
Do you need guidance in transforming your data landscape and prioritizing your data strategy for success? Learn how Xoriant Data and AI can help you modernize and integrate your data, enable advanced analytics and visualization, keep pace with data strategy trends 2024, and make the most of this treasure trove of insights for competitive advantage and long-term business success.