All Blogs
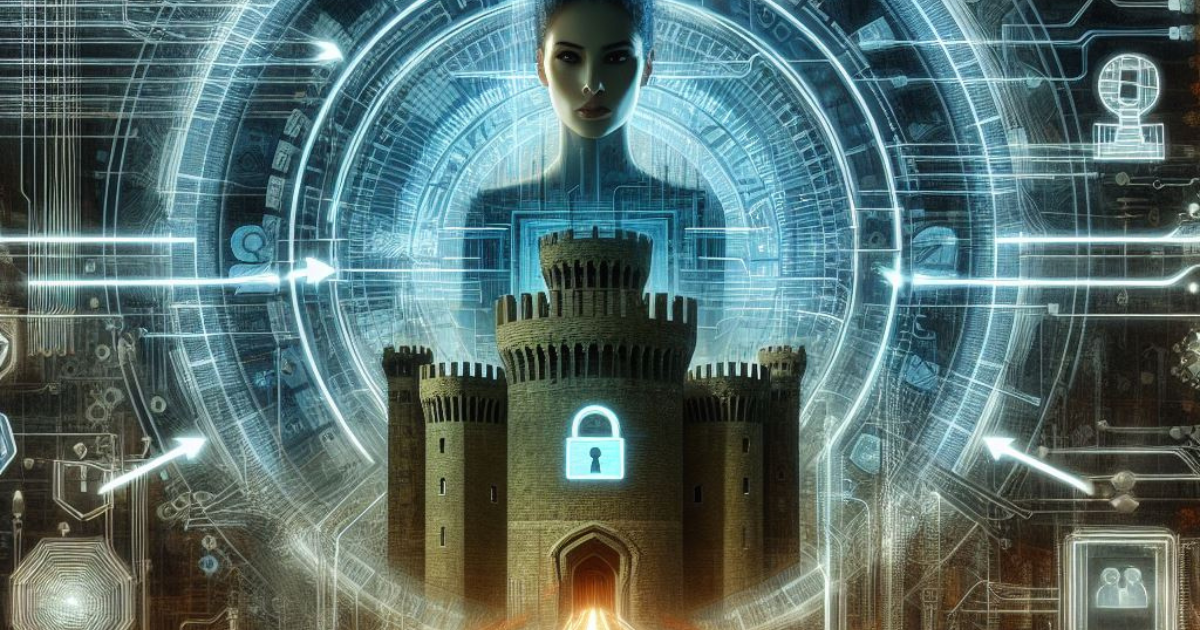
Zero Trust Security: A Cornerstone of Modern Cybersecurity
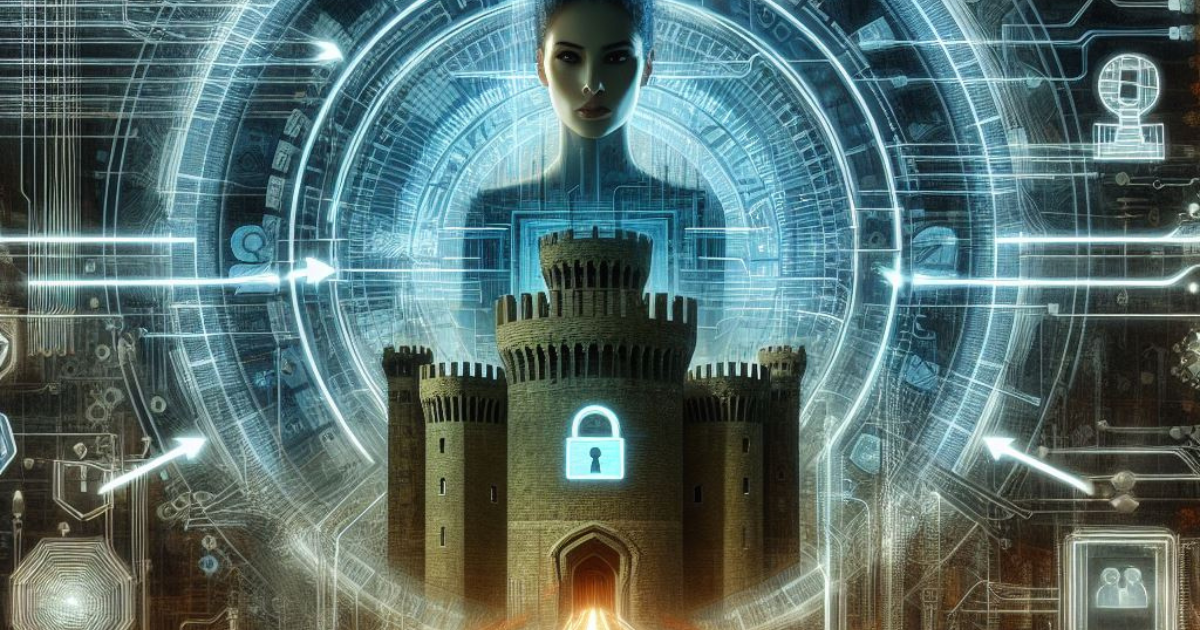
Zero Trust Security: A Cornerstone of Modern Cybersecurity
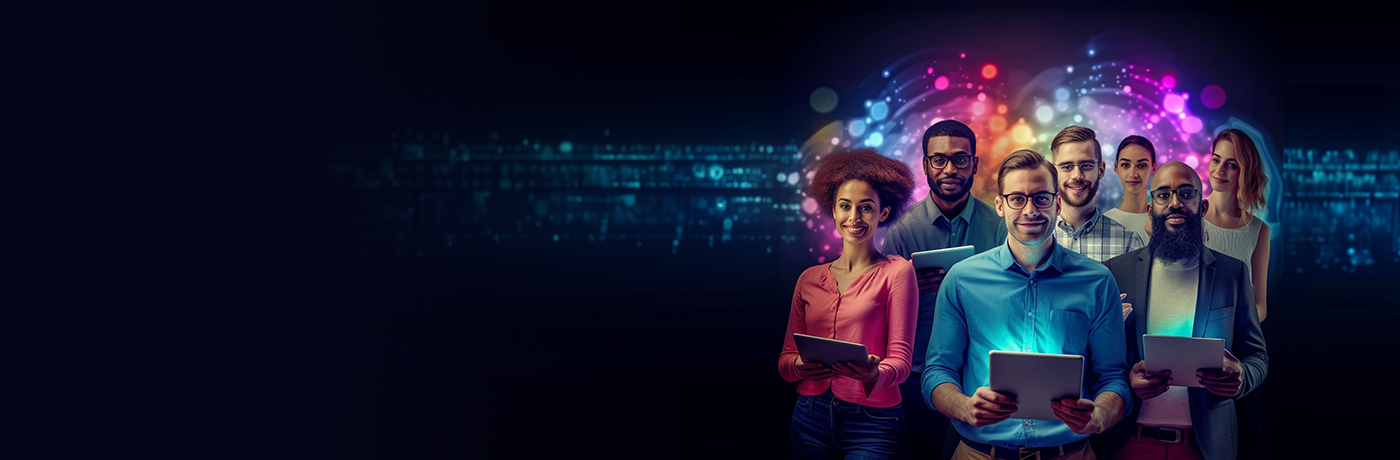
Talent Transformation is an Inside Job
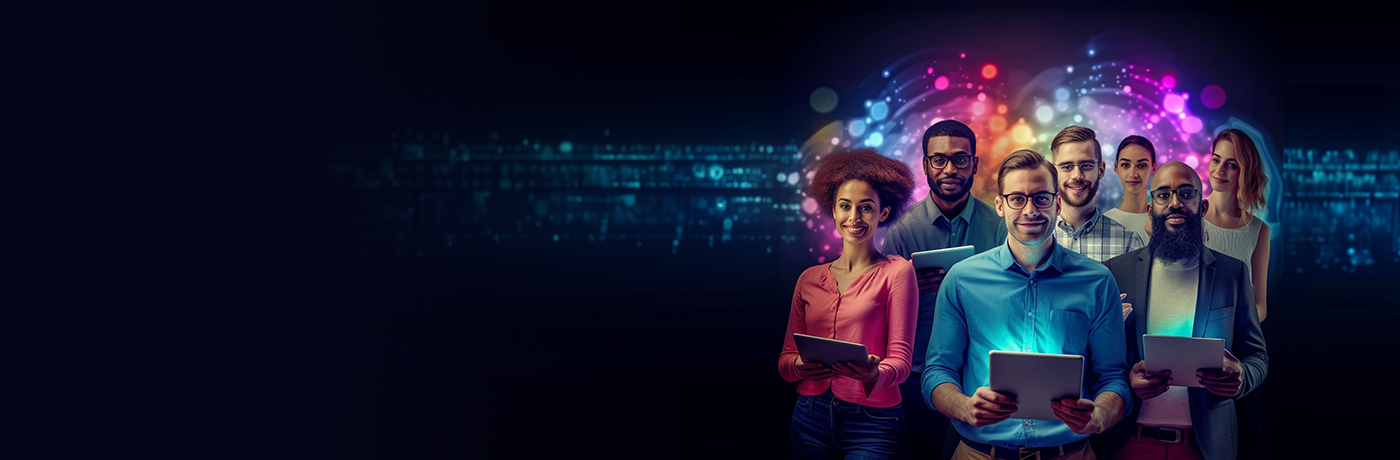
Talent Transformation is an Inside Job
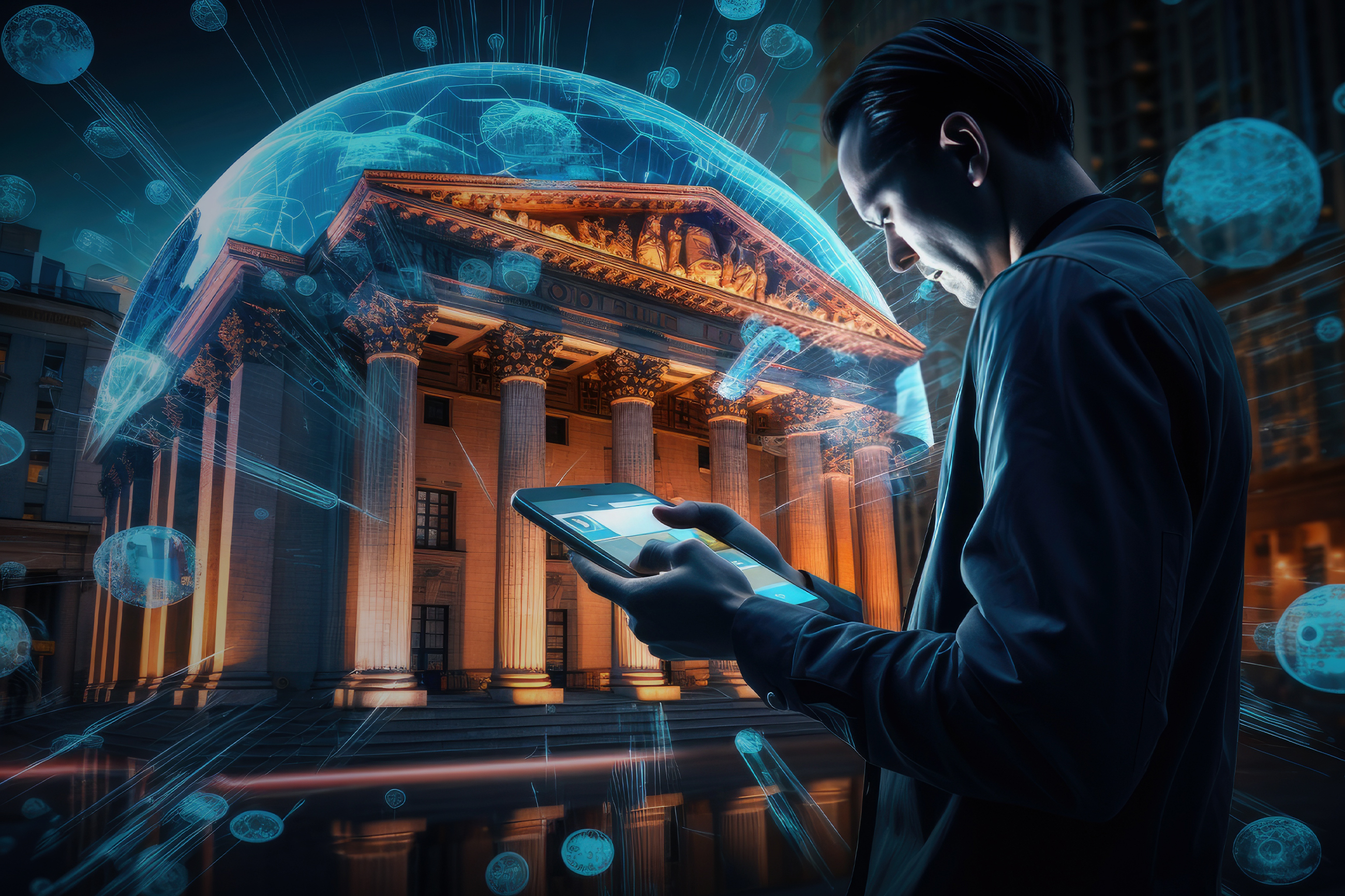
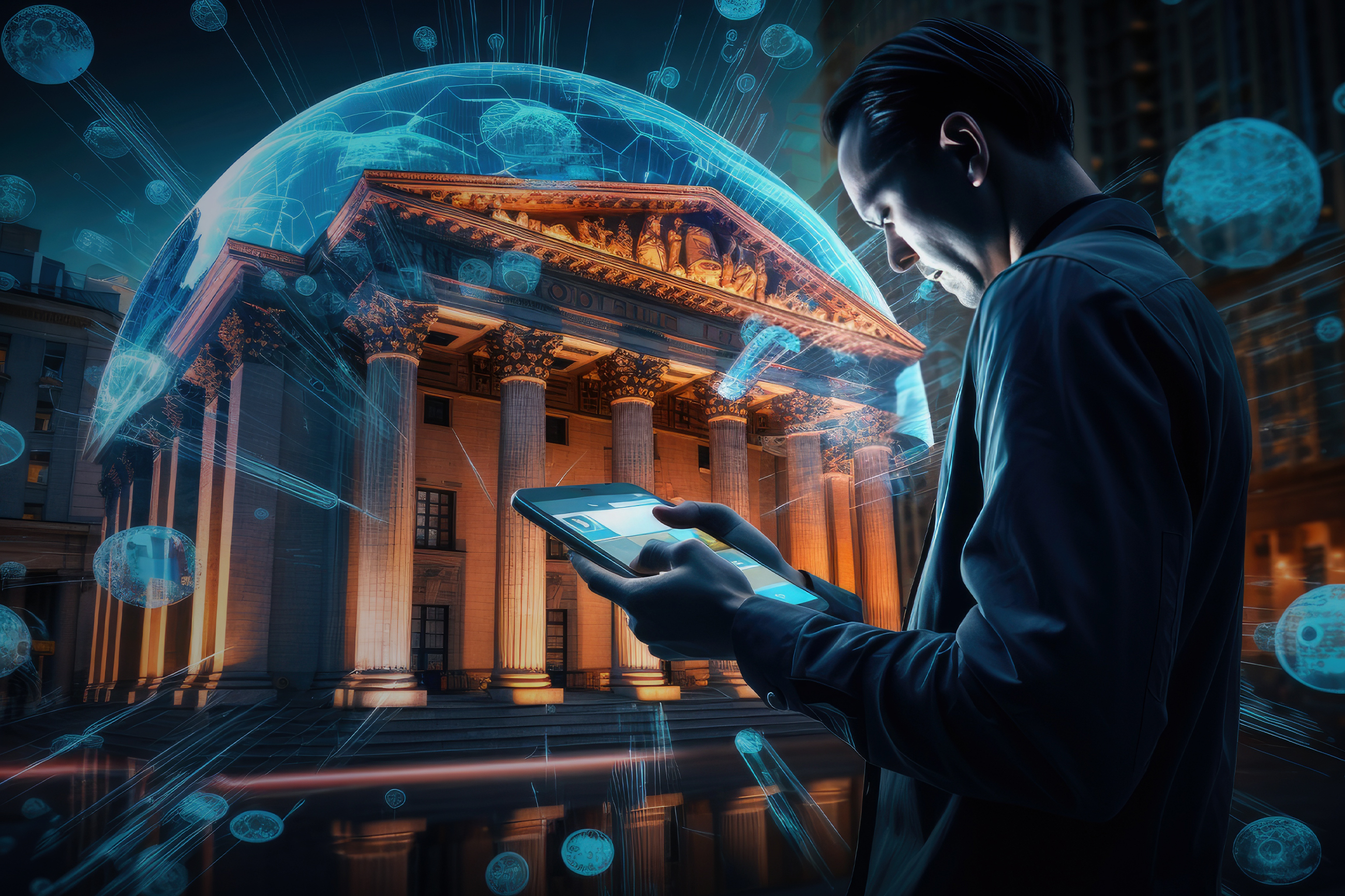
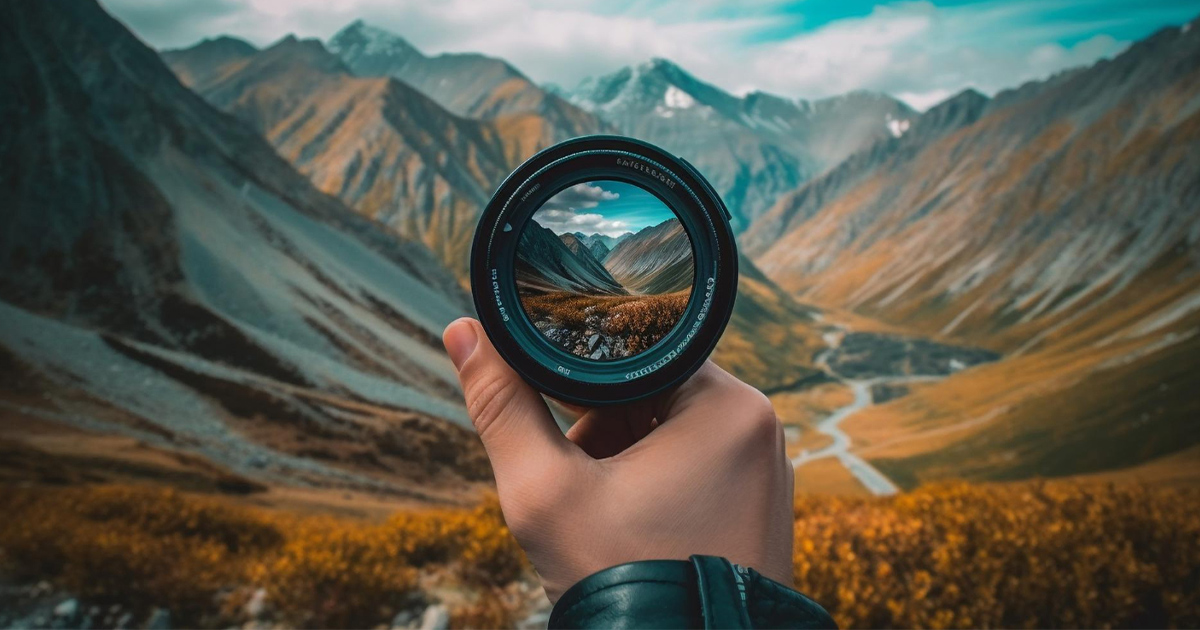
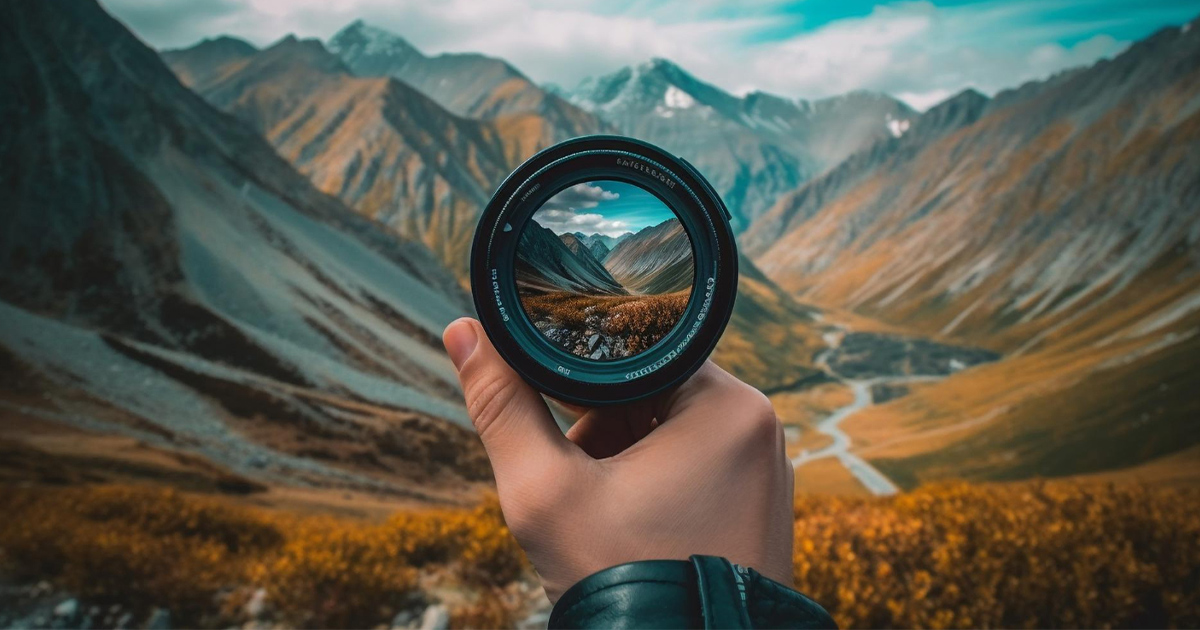
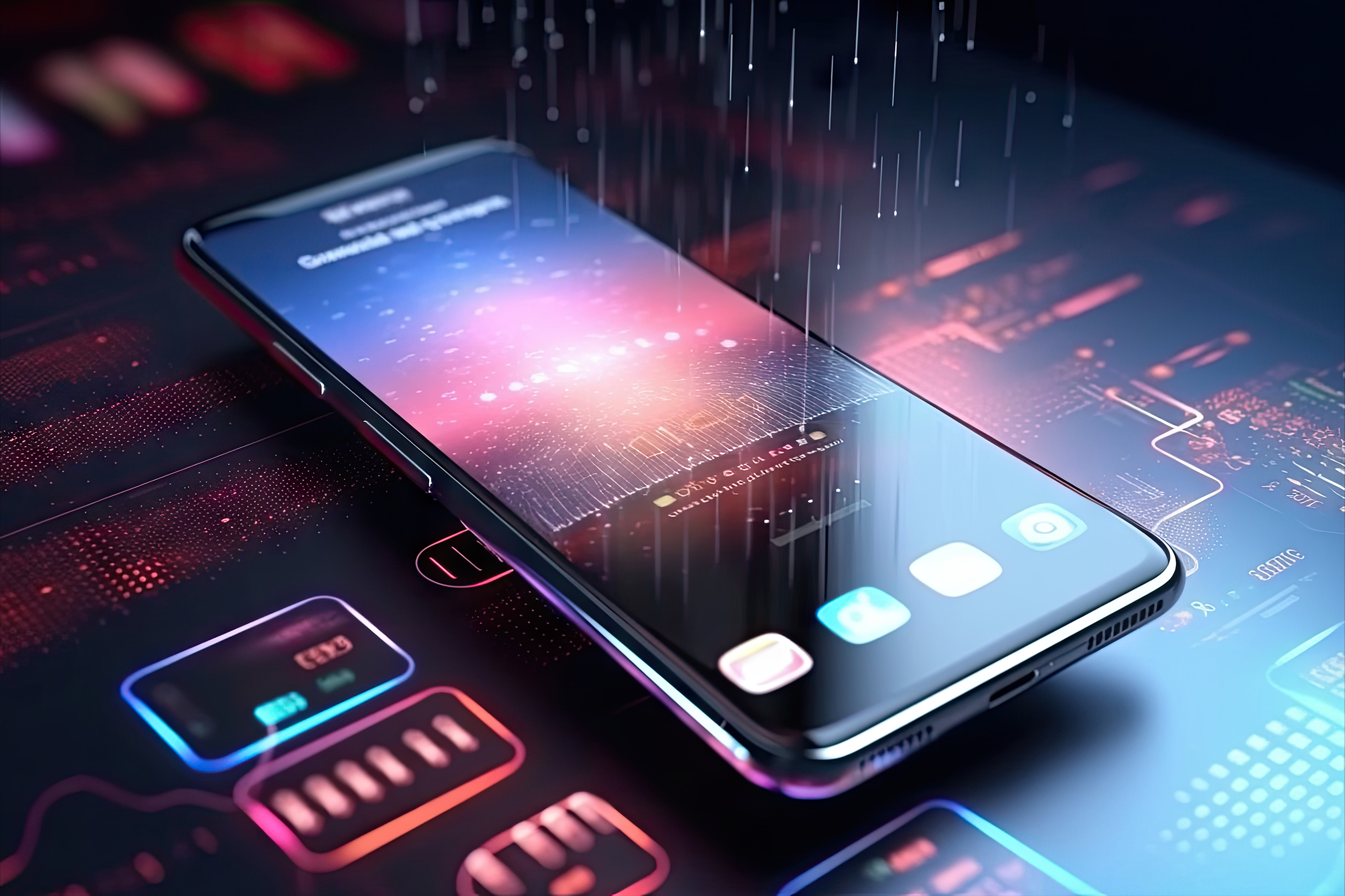
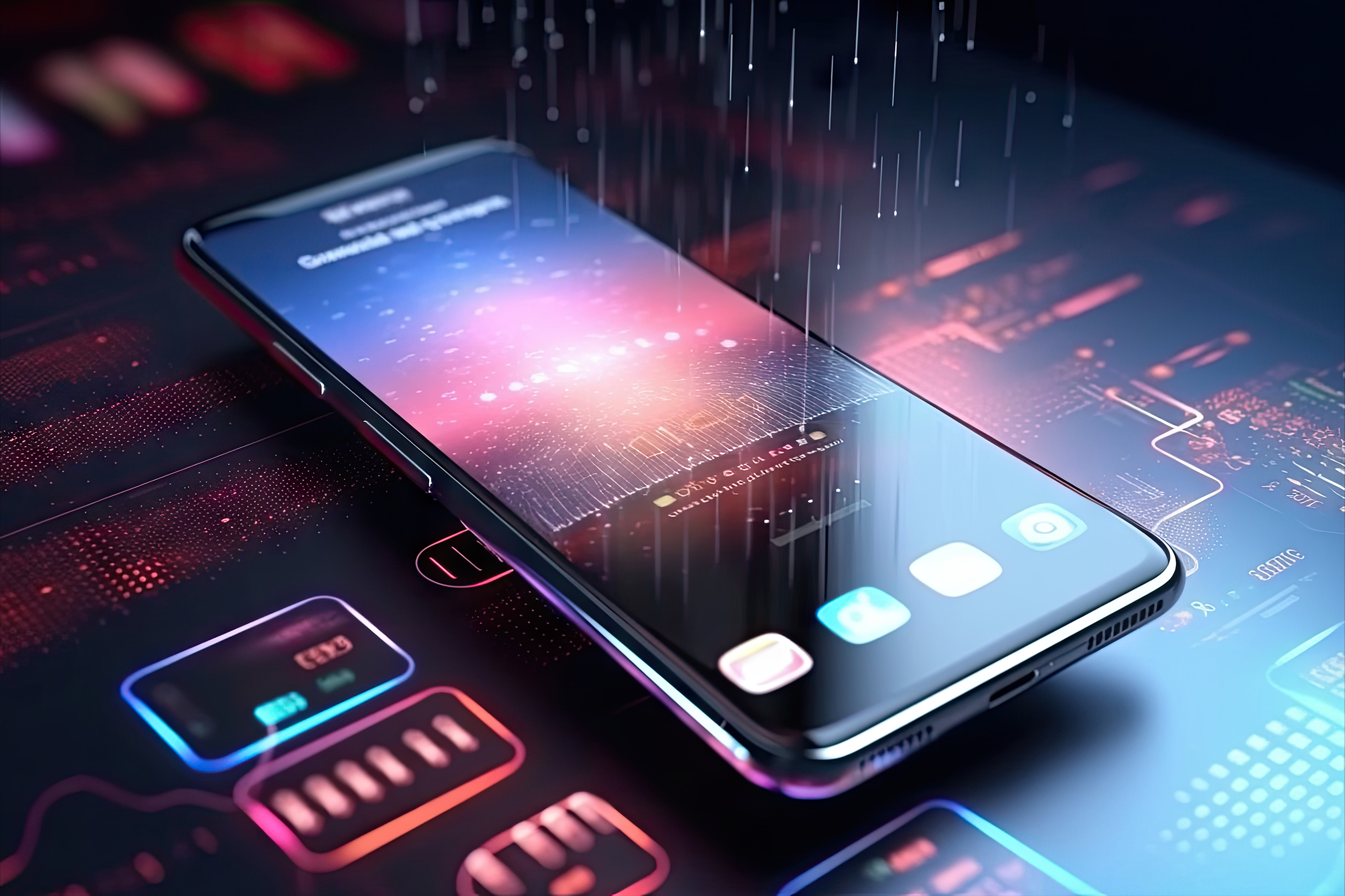

Channel Business Challenges To Desired Outcomes
Get In Touch With Xoriant

Think Tomorrow
With Xoriant
With Xoriant




